Manual and fragmented loan underwriting processes are becoming outdated in the digital era. Automating underwriting using data, algorithms and APIs is proving to be a game changer for lenders.
In this guide, we explore how Philippine lenders can implement automated underwriting to make faster, better credit decisions while reducing costs.
Why Automate Underwriting?
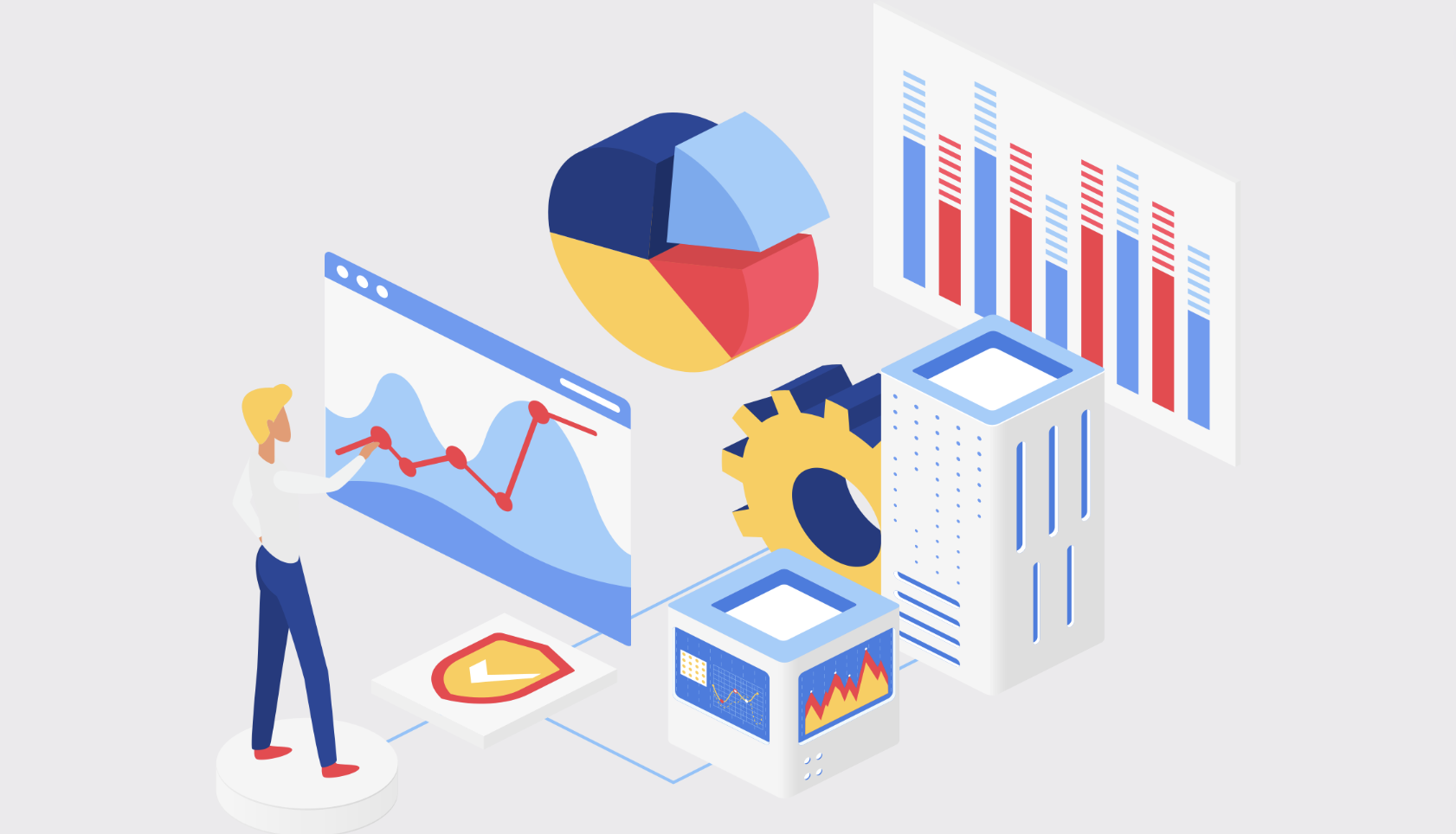
Here are some major benefits automated underwriting offers:
Faster Application Processing
Manual underwriting can take weeks, with extensive document verification and risk assessment delays. Automated systems slash turnaround times through instant data access and algorithmic approvals.
Improved Risk Modeling
Traditional scorecards are static and rely on limited variables. Automated underwriting allows dynamic risk models that can continuously improve over time. More data also enables better default predictions.
Lower Operational Costs
Manual underwriting requires extensive staffing. Automation cuts overheads through fewer document checks, reduced human errors and streamlined workflows.
Expanded Lending Reach
Traditional underwriting leaves thin-file segments underserved due to lack of bureau history. But alternative data allows assessment of gig workers, new-to-credit applicants and more.
Enhanced Borrower Experience
Manual underwriting often involves extensive paperwork and waiting. Automated real-time decisions provide a seamless experience for tech-savvy customers.
The ROI potential from automated underwriting makes it a must-have capability for lenders today.
Machine Learning for Credit Risk
At the core of automated underwriting are machine learning algorithms that can predict default risk and income volatility.
Machine learning models leverage large datasets to identify complex patterns between hundreds of variables. This allows more accurate credit decisions for both traditional and alternative applicant profiles.
For instance, neural networks can detect non-linear relationships and interdependencies between various attributes. This results in robust credit risk classification for thin-file consumers with minimal bureau history.
With automated underwriting, risk models continuously self-improve driven by the underlying algorithms. This creates a true competitive edge that keeps growing over time.
The Power of APIs and Real-Time Data
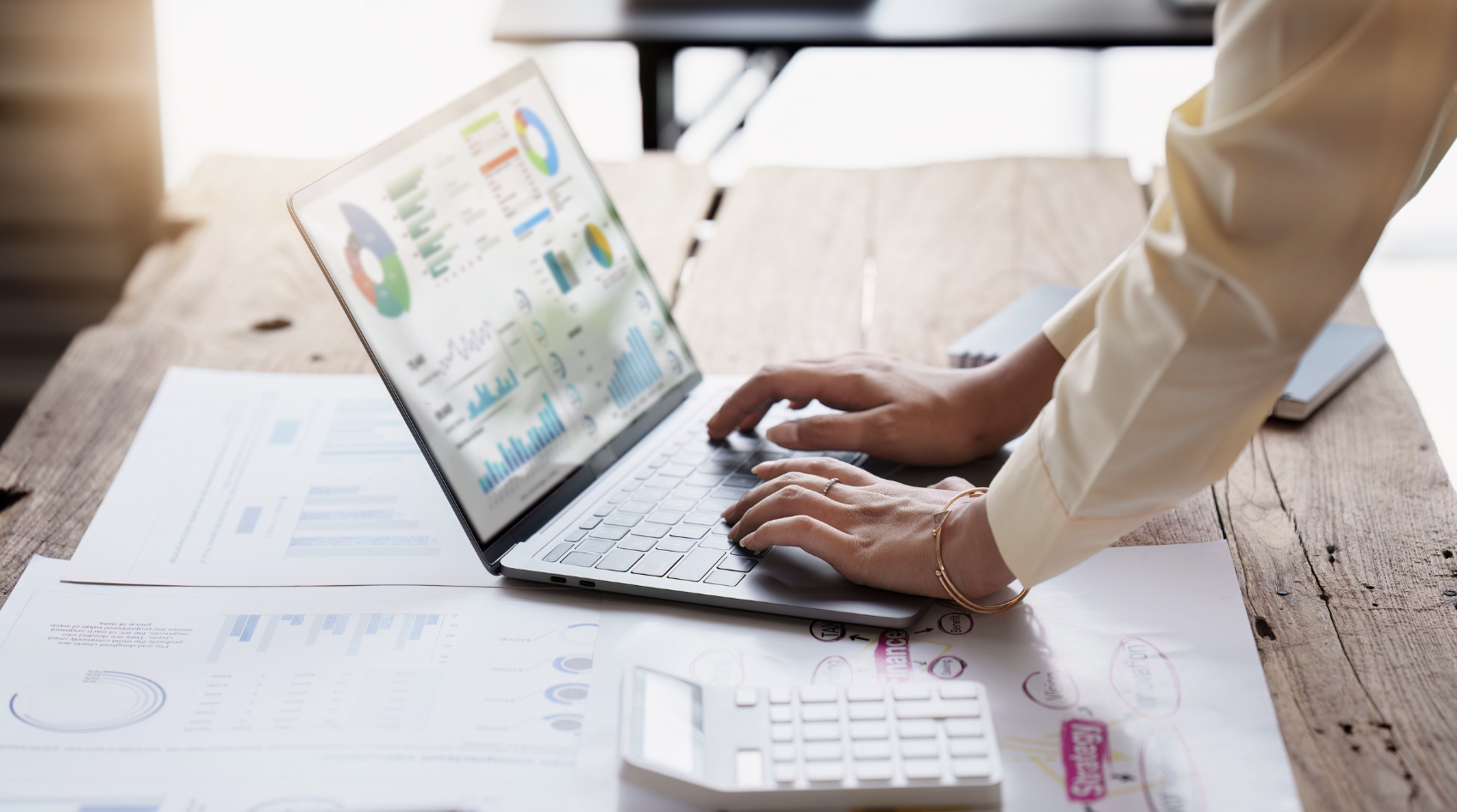
Automating underwriting requires seamless connectivity to multiple data sources from credit bureaus to government agencies.
Open APIs allow platforms to share information instantly based on user permissions. This removes friction and delays involved in manual data gathering.
Employment data APIs like Smile enable direct integration of payroll, tax and workforce data into underwriting engines. This provides real-time income verification capabilities missing in traditional systems.
As machine learning models become more sophisticated, the need for real-time, high-quality data increases. APIs are the enablers that allow underwriting automation at scale.
Reducing Manual Employment Verification
Verifying applicant employment history and income is crucial but time consuming in manual underwriting.
Automated verification through payroll and workforce data APIs eliminates paperwork and manual diligence. Lenders gain instant access to comprehensive payroll records and tax documents.
Applicants also benefit from paperless onboarding. Instant income checks using bank statement data further reduce document collection needs.
Employment data no longer needs to be a bottleneck holding back underwriting. APIs deliver it at the click of a button.
Steps to Implement Automated Underwriting
Here is a step-by-step approach to transform underwriting:
Assess Existing Models
Identify limitations in current scorecards and areas for improvement. Expanding thin-file approval rates is often a key goal.
Map Automation Opportunities
Find parts of underwriting workflows that can be automated through data connections. Income and identity checks are common early targets.
Onboard API providers
Start integrating employment, identity, bank statement and bureau API partners into the workflows.
Ingest Alternative Data
Feed diverse datasets like payroll, social media, geolocation and more into machine learning models to improve predictions.
Develop Risk Algorithms
Work with data scientists to build new ML-powered risk algorithms optimized for business goals.
Test and Iterate
Start with hybrid models using both manual and automated underwriting. Gather performance data, test across applicant segments and keep improving the models.
With the right approach, lenders can make the underwriting leap from legacy systems to fully automated AI-driven decisioning.
Conclusion
Automated underwriting is set to completely reshape lending. Solutions like Smile API provide the real-time employment and income data needed to enable automation at scale.
Contact us today to future-proof your underwriting. Our experts can help you map out an automation roadmap perfect for your goals, risk appetite and capabilities.
The opportunity to drive underwriting excellence through technology is here. Partner with us to lead the digital lending revolution in the Philippines.